Will GenAI Take Traditional AI Along for the Ride?
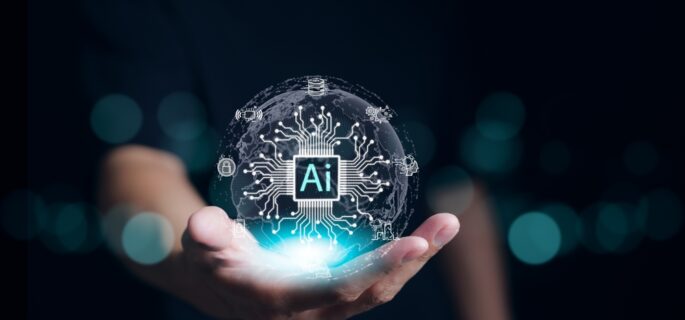
(SuPatMaN/Shutterstock)
Nearly a year after the launch of ChatGPT, companies now are falling over themselves in a rush to adopt generative AI to gain a new competitive advantage or prevent competitors from doing the same. But what about traditional forms of AI? Is there still room at the AI inn for good old machine learning?
So far, the rapid uptake of GenAI doesn’t appear to be causing any broad uplift of more traditional forms of AI, such as predictive models based on machine learning algorithms. At least that’s what the research is showing.
For instance, McKinsey’s recent state of AI report says 2023 has been GenAI’s “breakout year,” with one-third of organizations surveyed saying they already using GenAI regularly. What’s more, its survey suggests that 40% of organizations plan to increase their investment in AI overall because of advances in GenAI.
But there doesn’t appear to be any spillover into other forms of AI, McKinsey says.
“…[W]hile the use of GenAI might spur the adoption of other AI tools, we see few meaningful increases in organizations’ adoption of these technologies,” it wrote. “The percent of organizations adopting any AI tools has held steady since 2022, and adoption remains concentrated within a small number of business functions.”
A similar story is told by data coming out of the regular Fortune-Deloitte CEO survey. The summer edition of the survey, which was conducted in June, shows that 55% of CEOs were evaluating or experimenting with GenAI. However, only 39% of CEOs say they are evaluating or experimenting with predictive AI (although more CEOs said they were deploying predictive AI, 32% versus 13% for GenAI).
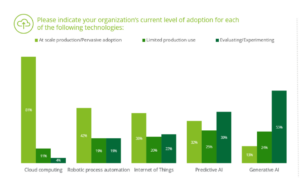
More than half of CEOs in June said they’re experimenting or evaluating GenAI (Source: Summer 2023 Fortune/Deloitte CEO Survey)
Those figures struck Forrester analyst Kjell Carlsson as interesting. “It’s just fascinating to see what attention lands on,” says Carlsson, who is now an AI strategist for Domino Data Lab.
Carlsson notes that there are meaningful differences in GenAI versus traditional AI. For example, GenAI is trained primarily on unstructured data, whereas traditional machine learning is largely based on structured data.
“All of a sudden, that world of unstructured data now becomes usable, and our challenge now is, what should we use it before?” Carlsson says. “We never used it before because it was too painful, and so we haven’t really built business models on the uses.”
The generative nature of GenAI is the real differentiator, he says. Many of Domino’s customers are using GenAI to develop internal assistants and chatbots that are based on the company’s internal corpuses of data, text, and reports. External chatbots that help with customer service are also popular. Carlsson is also seeing, surprisingly, uptake of GenAI among pharmaceutical companies using it to accelerate drug discovery.
But some things haven’t changed with the GenAI onslaught, especially when it comes to getting AI applications into production.
“All of those traditional capabilities that you needed around scale, around being able to incorporate the latest and greatest technologies, being able to go in and have observability and transparency around this, being able to go in and leverage hybrid cloud to do this easily and cost effectively–all of those become even more important in the realm of GenAI,” Carlsson says.
“Mysteriously, GenAI drives you right back to our original value proposition,” he continues. “We’d love to say that we had planned it that way. But it doesn’t mean that it isn’t true. It’s just one of those happy circumstances.”
While data science platform vendors like Domino are busy shifting their business models (if not their offerings) to target GenAI, there are other groups of vendors that are riding GenAI’s groundswell even higher.
OpenAI and its business partner Microsoft are leveraging the first-mover advantage to capture a significant share of the emerging GenAI market. Other vendors with foundation models, like Cohere and Anthropic, also appear to be doing quite well.
The market success of GenAI vendors reflects another important difference between GenAI and traditional AI: GenAI currently is largely something you buy whereas traditional AI is something you build.
This was the gist of a recent LinkedIn article by data and analytics consultant John Thomas. “Traditional AI models were mostly custom developed. In contrast, Generative AI applications are mostly built with vendor developed FMs,” he writes.
There are other important differences between GenAI and traditional AI projects, Thomas writes. For instance, getting started with GenAI requires smaller upfront development costs and can be stood up in a matter of days. Traditional AI, on the other hand, requires higher upfront costs and takes much longer to get started.
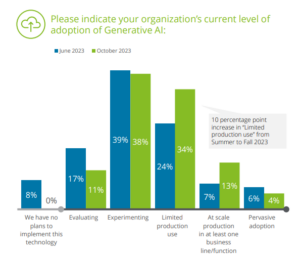
GenAI projects are moving quickly from the experimenting/evaluation phase to limited and full-scale production (Source: Fall 2023 Fortune/Deloitte CEO Survey)
The skills are also vastly different. In traditional AI, it requires skilled developers to create a model from scratch, as well as a lot of data preparation and data labeling work to train the model. But with GenAI, the models come pre-built and pre-trained. Instead, GenAI users focus much of their time on developing the right prompts.
With such large differences in technology, skill, costs, and data types, it’s no wonder that use cases are different, Thomas writes.
“Traditional AI uses are analytical in nature, involves predicting values or classifying observations, based on past data,” he writes. “Generative AI in contrast can generate content and perform tasks. The resulting use cases are fundamentally different and new capabilities include generation and manipulation of code, text, images, video, audio, and data.”
As organizations move from the experimentation phase of GenAI to the early deployment phase–which is already occurring, according to Fotune-Deloitte’s fall CEO survey–they will gain valuable knowledge around how to work with this technology. If past experiences with big data, machine learning, and traditional AI are any indication, there will be unexpected road bumps on the path to productivity (and that’s not even considering known GenAI concerns around hallucinations, privacy, and legal liability).
While the level of hype in the mainstream media would suggest that we have achieved the Holy Grail of AI–the creation of an artificial general intelligence (AGI)–those who are in the trenches of big data, advanced analytics, and AI realize that we are still far from achieving an AGI. What’s more, with less than a year of GenAI experience in most (but not all) organizations, the collective learning curve around GenAI is bound to be steep.
In the meantime, GenAI will continue to consume nearly all the oxygen in the room, at the expense of traditional AI. Once the sugar high around GenAI wears off and executives realize that it doesn’t offer a quick and easy path to transformational success while opening a host of new concerns around accuracy, transparency, and legal liability, then enterprises will find a firmer footing on which to do the hard but necessary work of integrating GenAI into existing IT stacks and with existing business models.
Editor's note: This article originally appeared on Datanami.
Related
Alex Woodie has written about IT as a technology journalist for more than a decade. He brings extensive experience from the IBM midrange marketplace, including topics such as servers, ERP applications, programming, databases, security, high availability, storage, business intelligence, cloud, and mobile enablement. He resides in the San Diego area.