Google’s Latest TPU v4 to Play Big Role in Google’s Growing ML Training Compute Services
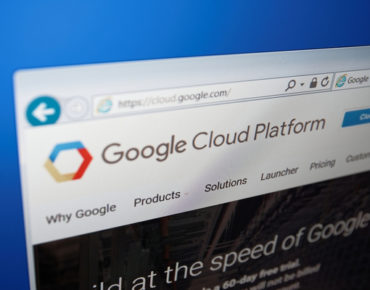
GOOGLE I/O 2021 – Google CEO Sundar Pichai spoke for only one minute and 42 seconds about the company’s latest TPU v4 Tensor Processing Units during his keynote at the Google I/O virtual conference this week, but it may have been the most important and awaited news from the event.
With the new TPUs, which are powered by Google’s latest v4 chip, the company has boosted the performance of its TPU hardware by more than two times over the previous TPU v3 chips, bringing critical new power and promise to machine learning training speeds on the Google Cloud Platform.
“Our compute infrastructure is how we drive and sustain these [AI and ML] advances and Tensor Processing Units are a big part of that,” said Pichai during the almost two-hour-long keynote on May 18 (Tuesday). “Today I'm excited to announce our next generation, the TPU v4. TPUs are connected together into supercomputers, called pods. A single v4 pod contains 4,096 v4 chips, and each pod has 10x the interconnect bandwidth per chip at scale, compared to any other networking technology.” [Pichai’s TPU comments begin at the 26-minute mark in the keynote].
The resulting computing power of the new TPUs means that one TPU pod of v4 chips can deliver more than one exaflop, or 10 to the 18th power, in floating point operations per second, said Pichai. “Think about it this way, if 10 million people were on their laptops right now, then all of those laptops put together would almost match the computing power of one exaflop.”
The new TPU v4 infrastructure, which will be available to Google Cloud customers later this year, is the fastest system ever deployed at Google, which Pichai called “a historic milestone for us.”
Creating an exaflop of computing power previously required a custom-built supercomputer, he said. “But we already have many of these deployed today, and we'll soon have dozens of TPU v4 pods in our data centers, many of which will be operating at or near 90 percent carbon-free energy. It's tremendously exciting to see this pace of innovation.”
Google’s previous version TPU 3.0 was unveiled in 2018.
TPUs are Google's custom-developed application-specific integrated circuits (ASICs) which are used to accelerate ML workloads. Developers can use Google Cloud TPUs and Google’s TensorFlow open source machine learning software library to run their ML workloads. TensorFlow was developed and first released by Google in 2015.
Google Cloud TPU is designed to help researchers, developers and businesses build TensorFlow compute clusters that can use CPUs, GPUs and TPUs as needed. TensorFlow APIs allow users to run replicated models on Cloud TPU hardware, while TensorFlow applications can access TPU nodes from containers, instances or services on Google Cloud.
Several AI analysts were quick to tout the TPU v4 news and what it will mean for enterprises that are faced with constantly growing ML training demands.
“If you’re trying to train a large AI/ML system, and you are using Google’s TensorFlow specifically, this will be a big deal,” Jack E. Gold, president and principal analyst with J. Gold Associates, told EnterpriseAI. “There is never enough processing power when large models are being trained, with some taking days or weeks to run on current systems available in the cloud, and mostly based on highly parallel GPUs. And this can be very costly in terms of cloud costs and power.”
What Google has done in response with its TPUs is to build chips that are highly optimized for TensorFlow-based modeling to expedite the training of models, especially those that must be updated often or that use large data sets, said Gold.
“So, what Google is doing here with its v4 chip is to dramatically increase the compute horsepower available, and reduce time to model significantly,” said Gold. “They are also enabling much larger models to run in a reasonable amount of time. But equally importantly they are reducing the amount of power per model – since if the models run faster, they use less total power. And that’s also good for their cloud data centers costs, as well as just sheer capacity to handle more users.”
And by using Google’s own TPUs, this is also a move by the company to continue to substitute its own processors for those of other vendors, he said. “Google wants to stay ahead of the others like AWS and Microsoft, that are also building their own accelerators for their AI cloud-based services.”
Gold also noted that since Google does a lot of its own AI/ML/DL modeling that anything the company can do to enhance its own internal needs with additional capabilities is a big win for them. “It’s not just about supporting external customers – it’s also about their own requirements,” he said.
Charles King, principal analyst with Pund-IT, said that Google’s ability to double the performance of the previous v3 chips while also achieving exascale performance in a single V4 pod are both impressive.
“It's a notable achievement that demonstrates the company's technical acumen and its willingness to continue funding chip development,” said King. It’s also important for the company’s customers, he added.
“Absolutely, since these new chips will be powering AI-related workloads and services offered in Google Cloud,” said King. “If Google can deliver superior performance at highly competitive prices, it could diminish the value of competitors' services.”
Holger Mueller, principal analyst at Constellation Research, said the TPU v4 news was “one of the most exciting announcements of Google I/O … as the company builds out its lead with algorithms on silicon with TPU v4.”
With this development, Google keeps building its lead on AI compute over AWS and Microsoft Azure, Mueller said. “[This is the] first architecture to reach an exaflop – and AI needs it. When you do it Google-style… the faster and cheaper AI will win in business and government, including with the military.”
Another analyst, Karl Freund, founder and principal analyst for machine learning, HPC and AI with Cambrian AI Research, said that early benchmarks are promising for the new TPUs.
“TPU v4 looks like a winner, based on early MLPerf benchmarks,” said Freund. “We await final benchmarks which I expect to see this summer when we get closer to the announcement of availability and pricing later this year. It has been a much longer time coming compared to earlier TPUs but may well be worth the wait.”