Deep Learning Boosts Call Center Speech Recognition During the COVID-19 Crisis
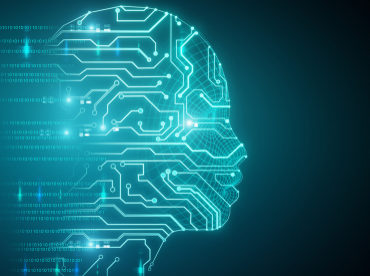
A business operation hard hit by COVID-19 is the call center. Industries ranging from airlines to retailers to financial institutions have been bombarded with calls—forcing them to put customers on hold for hours at a time or send them straight to voicemail.
Data reveals the picture. A recent study from Tethr of roughly 1 million customer service calls showed that in just two weeks, companies saw the percentage of calls scored as “difficult” double from 10 percent to more than 20 percent. Issues stemming from COVID-19—such as travel cancellations and gym membership disputes—have also raised customer anxiety, making call center representatives’ jobs that much more challenging.
Companies thinking about investing in speech recognition should consider a deep learning-based approach, and what to take into consideration before implementing it.
Accuracy and Efficiency
To convert audio to text, traditional speech recognition methods must first convert audio into phonemes, which are reassembled into predicted words to generate transcripts. It’s a complex and convoluted process that takes time, forgoes context entirely and delivers lower accuracy.
But an end-to-end deep learning approach uses an optimized CNN (Convolutional Neural Networks)/RNN (Recurrent Neural Networks) hybrid model trained on GPUs. It’s optimized to deliver better accuracy under real-world conditions.
As enterprises adapt to an entirely remote workforce during the pandemic, they’ll need to especially arm call center and sales teams with the right resources to ensure a seamless transition—including better training, communication around best practices, and streamlined processes. Accurate ASR (automatic speech recognition) tools can help bridge the gap by providing an accurate call transcript, analyzing the main talking points, and revealing areas where employees can improve to boost customer satisfaction overall. To implement a speech recognition model, building more data centers isn’t the right solution—it’s extremely expensive and they’ve become too big and slow instead of more cost-effective and efficient.
But a deep learning-based approach allows enterprises to pick which pieces of the puzzle to build the model from, and then train the model to build itself. In many cases, 10 hours of thoughtfully selected audio is all that’s needed to effectively train a model. By doing the work up front, the model can continue to optimize its performance over time, and companies can extract more accuracy and scale out of it. When your enterprise is ready to invest in deep learning, here are a few things you need to implement and consider:
Standardized Tests
Once companies have the right resources and enough data, they should set up standardized tests to compare word error rates in outputs. Doing this means curating 100 segments of audio from random files of customer service calls with each segment a minute long, and then get those files labeled by a service such as TranscribeMe for about $100. A good standardized test will represent real-world data and include complex conditions – with background noise, multiple speakers, diverse accents, different topics – to give you a great idea of whether a model will be strong or weak.
For example, if you want to custom-train your model to accurately capture meetings to identify insights and trends, consider inputting examples of real meetings into the system for it to effectively understand the speech dynamics and nuances. For example, imagine being able to easily identify questions or concerns that come up in sales conversations, so your sales team can better anticipate and answer those concerns. Inputting an audiobook, for example, would not be as effective, as the model would only learn from one voice and be unfamiliar with participant interruptions or background noise, which commonly happens during conference calls. Using real-world audio for these standardized tests will serve your company better.
Don’t Ask the Impossible
If you’re looking at your training data and can’t learn the task at hand (such as comprehending a conference call with zero errors), then it doesn’t matter how good of a model builder you are, your speech recognition tool likely won’t be able to learn it either. That is, don’t let yourself believe that the deep learning magic black box can learn anything if you throw enough data at it. To ensure success, be sure that you’re taking the necessary steps to determine if this is a winnable battle. For example, you can ask yourself: could a human do this with a second’s worth of thought and come out with a reliable answer? If so, it’s likely something a machine could do as well.
Pairing Custom Training with End-to-end Deep Learning
As we embrace our new normal, enterprises of all sizes are regularly evaluating which processes are working and which need to be improved or eliminated. Now that workforces are distributed and best practices need to be shared remotely, it’s especially critical to have the right speech recognition software to streamline communication efforts across teams, identify trends, and unlock business value.
Although speech recognition has come a long way in the last few years, much of it is still only optimized for one-way, short-form conversations like those delivered to an assistant. A custom-trained model with an end-to-end deep learning approach can reach a 90 percent accuracy rate or higher. Looking a few years ahead, I believe enterprises will attain 95 percent accuracy with less data or even no data at all, as their systems will have their muscles built up enough for that level of accuracy.
Dr. Scott Stephenson is CEO and co-founder of Deepgram, a speech recognition company.