AI Agent Provides Rationale Behind Decisions
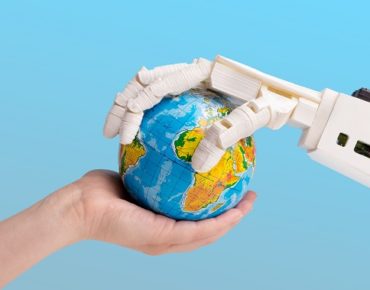
Frameworks for explaining the rationales underlying AI-based decisions continue to make progress in the form of an AI agent that attempts to provide straightforward English explanations in a readily interpretable form. Eventually, users could seek alternative explanations. (See also Explaining AI Decisions to Your Customers: IBM Toolkit for Algorithm Accountability.)
University researchers tackled the explainable AI dilemma by training an AI agent to play a video game and explain the reasoning behind each of its moves. They also trained their “neural rationale generator” to come up with different styles of reasoning, then gauged how humans perceived various explanations.
Among the goals is building trust among human-AI teams by developing an AI agent capable of delivering readily understandable explanations that could eventually be used by non-experts.
The AI agent experiment was conducted by researchers from Georgia Tech, Cornell University and the University of Kentucky. They used a video game called Frogger to train an AI agent to generate explanations for various moves and strategy.
A pair of user studies sought to establish whether the rationales generated by the AI agent made sense to humans in terms of clarity and logical justifications for various video game moves. Then the investigators explored user preferences among different styles, attempting to gauge the level of on confidence in the AI agent’s explanations and where machine-human communications broke down.
“We find alignment between the intended differences in features of the generated rationales and the perceived differences by users,” the researchers reported in their automated rationale generation study published earlier this year.
“Moreover, context permitting, participants preferred detailed rationales to form a stable mental model of the agent's behavior,” they added.
The researchers noted that their AI agent represents “formative step” toward understanding how humans perceive attempts to explain the rationale behind AI-based decisions. “Just like human teams are more effective when team members are transparent and can understand the rationale behind each other’s decisions or opinions, human-AI teams will be more effective if AI systems explain themselves and are somewhat interpretable to a human,” Devi Parikh, an assistant professor at Georgia Tech’s School of Interactive Computing, told the Association for Computing Machinery (ACM).
The AI agent is part of growing field of inquiry into explainable AI and the growing need—especially among enterprise users—for frameworks used to explain the rationales behind the decisions made by machine learning and other models. For instance, IBM researchers just released a toolkit of algorithms aimed at interpreting and explaining those models.
The IBM effort reflects survey results that found more than two-thirds of business executives want greater AI explainability.
Meanwhile, the university researchers said their rationale generation framework includes a methodology for collecting explainable AI building blocks like high-quality human explanation data. These data can then be used to configure neural translation models to produce either specific or general rationales for decisions.
The researchers also sought to quantify the perceived quality of the rationales, and which were preferred by human users.
They told ACM they are mindful of the need to “keep issues of fairness, accountability, and transparency in mind first before thinking of commercialization” of the AI agent technology.
Future research would focus on adding interactivity to the AI agent, enabling users to question a rationale or ask the agent to provide an alternative explanation. That ability to reject and explanation has previously been shown to boost user confidence.
Related
George Leopold has written about science and technology for more than 30 years, focusing on electronics and aerospace technology. He previously served as executive editor of Electronic Engineering Times. Leopold is the author of "Calculated Risk: The Supersonic Life and Times of Gus Grissom" (Purdue University Press, 2016).