AI Pitfalls and the Hidden Value of the ‘Citizen Data Scientist’
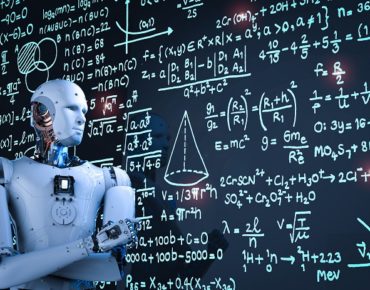
Buzzy new technologies are commonly perceived, at least for a while, as panaceas. When Big Data emerged some years ago, Hadoop hype was rampant. Addison Snell, CEO of industry watcher Intersect360, jokes about data analytics project managers viewing Hadoop as something of a magic potion. “There was this big notion of ‘Hey, I've got this data, let's get a jar of Hadoop and rub it on our data… What do you mean, we still have big data problems? Did we run out of Hadoop? Get another jar!’”
Today, as more enterprises pursue machine/deep learning explorations, hype rages around new AI tools and the wizardry of data scientists. But both of them have potential (and related) shortcomings that can damage enterprise AI strategies, particularly in the early stages.
How organizations should go about getting into AI was a core topic of discussion by a panel of AI practitioners at Tabor Communication’s recent Advanced Scale Forum. Over the course of the conversation, a divide emerged: On one side are new AI tools and data scientists who have expertise in building AI models; on the other side is the data possessed by an organization combined with in-house staff knowledge of that data and of the organization’s business. The value of the former's shiny-new-object can be inflated, the latter's value can be overlooked.
In his introductory remarks, Snell noted that his firm’s research indicates that more than half of organizations with HPC infrastructures are engaged in ML types of initiatives while “most of the rest are planning on it.”
At this nascent stage of AI, he said, the first consideration shouldn’t be which cool new tool to use but what you’re trying to do with it, and whether you’ve picked the right tool based on the project’s objectives. Drawing an analogy, Snell said, “It doesn’t take a lot for me to get a table saw. I can get started with it. But ownership of the table saw is only one part of whether I'm going to do something constructive with it. I can cut up a lot of wood, but for what purpose?”
Shane Brauner, VP of IT and operations at Schrödinger, maker of chemical simulation software used by pharmaceutical, biotechnology and materials science companies, agreed.
“For us, it doesn’t take a lot to get started with the tools themselves,” Brauner said, “but understanding what's going on behind the data itself, behind the tools itself, understanding what you're looking for rather than just expecting this to be a silver bullet,” is important.
Brauner emphasized the value of the company’s data in Schrödinger’s machine learning project work.
“We're a bit lucky in the sense that we get to ‘dog food’ our own products,” he said. “Classically, Schrödinger has built tools for improving drug discovery. So a lot of them are physics-based simulations. And those are able to actually provide new data into our ML models. So as far as getting started goes, understanding what you’re going for, what you’re looking for, and having a way to measure that, is really critical.”
HPE AI Evangelist Steve Heibein argued that data is the real gold.
“Data is intellectual property today, it's not the models,” he said. “In fact, Google and others give away the models, but they keep the data… So it’s really what your data is, but obviously in any of these projects, it's what's the goal? What do you want to get out at the other end?”
Jay Kruempke, senior product manager at enterprise open source-based software vendor SUSE, said your data, your expectations of it and flexibility in what you do with it are the fundamentals for successful ML projects
“Number one, you have to know what you’re attempting to accomplish,” he said, “so you start out with a goal in mind: what do I think the data is going to reveal for me? But you have to be willing to understand that as you dig into it you're going to have to iterate, because you may find out things that you didn't know that might be a better direction than what you originally stated out with."
As the project work matures, business managers will start to see the value, “they’ll say, ‘Hey this is good stuff and we can afford to invest more in it,’” Kruempke said. “So start small, iterate, keep going after it.”
That comment echoes the mantra heard at IBM’s annual Think conference earlier this year; when it comes to the beginning stages of implementing AI: “start small, think big, move fast.”
This brings us to data scientists, those sought-after unicorns with “kitchen sink” skill sets(see “Data Science in a Box: Tools Attack Critical Skills Shortage”) and their proper role for companies starting out with machine learning.
Said Wayne Rickard, chief strategy officer at in-memory computing company Formulus Black, “What a lot of people do when they start up a machine learning initiative or an AI initiative is think, ‘I've got to go out and hire the smartest Ph.D. data scientist that I can find.’ You bring the guy on board and he doesn’t understand the business processes…, he doesn't understand the end goal. So it's really important to bring the business process people in early so that they can talk about the type of business objectives and put it in the language of the business and not just focus on being able to manipulate the tools.”
This isn’t to disparage the value of data scientists, it’s to emphasize that new tools and data scientists alone can only get you so far. It points to the importance of existing, in-house knowledge of the organization’s data and its business – both of which, no matter how brilliant the newly hired data scientist is, take time to learn.
HPE’s Heibein used the term “citizen data scientist” for “people who already know your data, they understand it, they've been working with it, they've been part of your organizations for a while. Citizen data scientists are those people who don't have the Ph.D.’s., they're not formally trained, but they’re the ones who may be doing your business intelligence work within your corporations your organizations.”
He added that AI democratization tools like DataRobot, H2Oai and SparkCognition give citizen data scientists “guardrails” to help them “take your data, run it and apply it against different machine learning and deep learning models…to actually get information out the other end. These are quick ways to get to a proof-of-concept. And at that point you can get more funding and move forward.”
SUSE’s Kruempke discussed the wisdom of data scientists and their “citizen” counterparts working in tandem.
“I think it's certainly smart to invest with the people who already know your business,” he said, “that have at least some of the idea of the problems and the data that's available. If you bring somebody from outside, they're not going to have that background. But on the other hand it's also good to have at least some subject matter experts, so these citizen data scientists don’t pound their heads against the wall. You need a mixture. You certainly don’t want to go out and try to outsource the whole thing. But you need to have people who can be a trusted resource as you kind of work toward what the end solution looks like.”
Schrödinger’s Brauner agreed. “It's both. You can't just bring somebody in and expect ‘that’s that.’ It's a continual reinvestment where, if you bring people in from the outside, they need to learn your business. If you've got people who know your business, they need to learn the tools. So I think it's a continuous evolution as you go through it.”