How to Avoid an AI Winter and Kick-start the AI Renaissance
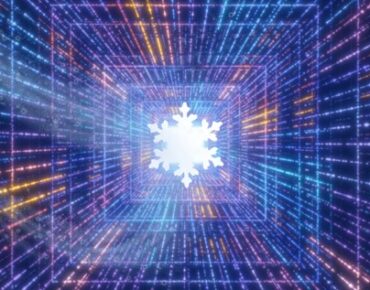
Generative AI solutions like ChatGPT and Bard are both exciting and impressive in their ability to take in enormous datasets and build complex correlations to generate content from just a simple prompt. The massive advances realized by these approaches are an indicator that the field of artificial intelligence is on the verge of a renaissance. The trouble is that neither ChatGPT nor Bard are able to check the accuracy of their answers and, let’s face it, there’s a huge difference between sounding correct and actually performing well. As a result, chatbots are not actually useful for solving business problems.
If this disconnect sounds familiar, it’s because we’ve been at this exciting technological precipice before. The 1950s saw the computational boom that led to the coining of the term “artificial intelligence” itself, and the 1980s saw breakthroughs in neural networks and the personal computing revolution that enabled widespread access to computation. But at both of those junctures, AI never met the lofty expectations generated from the hype. The subsequent disappointment and disillusionment with the technology led to a stagnation in research funding and a decline in the development of artificial intelligence technologies. The result was the beginning of the "AI Winter."
To avoid another stagnation, it is important to acknowledge the real limitations of generative AIs and address these limitations by developing and strengthening other key AI technologies.
How Generative AIs Work
Generative AIs use large language models, which are neural networks trained on a vast amount of text. Their power comes from their ability to build correlations on these massive datasets. Once the AI has been trained on sufficient data, if given a short prompt, it can then recall the style of the text it has seen before, and generate content with a similar design.
While the technological prowess these AIs demonstrate carries the same excitement as the development of web browsers in the 1990s, there are different beliefs as to how to move the field forward. Some believe that generative AIs can solve any problem, given enough data; their path towards an AI renaissance simply requires training generative models on more data. But this is fundamentally ignorant of the fact that there are entire classes of problems that generative AIs simply cannot address.
Buyer Beware: Understanding the Current Limitations of Generative AIs
Despite the interest in ChatGPT and Bard, users/and scholars both indicate that there is a huge, and consequential, difference between sounding correct and truly performing well. Sure, generative AIs are producing answers to prompts that sound impressive, but they are not necessarily accurate. This is because they are not designed to understand if their answers are correct and there’s a wide swath of problems where accuracy is of the utmost importance. The problem is that traditional generative AIs are trained on generic models using an abundance of unvetted data and they are incapable of leveraging a business’ internal data. Though internal data is necessary to answer some of the most urgent problems, generative AIs are unlikely to get access anytime soon as sensitive customer data would not be public on the internet, and generative AIs are not constructed to learn from smaller, “business-sized” data.
As Noam Chomsky points out, another example of ChatGPT’s limitations involves the concept of causation. For instance, if a person asks a question such as “If it rains, will the grass be wet?” intuitively, that person would understand that the rain causes the grass to be wet. Yet, ChatGPT is not programmed to have a sense of causality, to understand the hierarchy of knowledge that underpins a logical sense of cause-and-effect. Another often overlooked limitation of ChatGPT involves the computing costs needed to support it. Each subsequent generation of generative AI requires major increases to compute costs as it needs to intake more and more information to improve its correlative approaches making it prohibitively expensive for most organizations.
Alternative Technologies Poised to Jump-start the AI Renaissance
For AIs to become truly powerful in solving a wide class of problems, other technologies will need to develop concurrently with generative AI. Combining a variety of modern technologies can compound gains in their ability and guide AI into a renaissance. These include:
- Causal AI which is designed to determine cause and effect and control for other variables. It’s standard industry practice to use A/B testing to figure out what intervention—e.g. “What sized promotional discount should I be sending my customers?” — and determine causes distinct to an individual customer’s behaviors. Running A/B testing at massive scales, with many different simultaneous variables, is tricky—and here is where the power of causal AIs can be harnessed. Correlative AIs alone cannot understand the logical chain reactions that underpin the reasons why, if it is raining, a person may not want to have a picnic.
- Online Learning is a technical term for an AI that continuously updates itself based on conditions, predictions, and accuracy. Current generative AIs are unable to update themselves based on feedback in real time. Consider the pandemic. Consumer habits change drastically and no amount of historical data could have predicted how buyer behavior would change and how organizations would need to pivot. Bottom line is any AI worth its salt needs to be nimble enough to learn “on the ground,” in real time, and provide answers based on actual conditions to solve the relevant problem.
With this combination of technologies, AI can tackle a myriad of different classes of problems. Notably, while AI has ordinarily worked with massive datasets, having an “AI Swiss Army Knife” can enable decision making on the scale of individual datapoints and limited datasets like the transactions of a single business. More importantly, it could be the start of a true AI renaissance that the world has been waiting for.
About the Author
Anthony Chong is CEO/Co-Founder of IKASI, providers of sophisticated, predictive AI solutions that help organizations drive profits. To learn more, visit them, connect on LinkedIn, or follow them on Twitter.