Spell Bringing MLOps to Deep Learning to Ease the Deep Learning Path for Enterprises
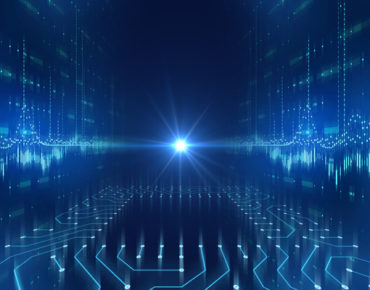
Making machine learning operations easier to use, manage and organize for enterprises has always been the goal of the series of best practices known as MLOps.
But while MLOps works well for the needed processes and commodity CPU-based infrastructure of traditional machine learning, it can come up short in being as useful for more complex deep learning workloads, which can be far larger and more demanding than traditional machine learning requirements.
To fill this gap, New York-based startup, Spell, has launched what it calls a cloud-agnostic MLOps platform that is targeted to serve the more complex and unique needs of deep learning using the principles of MLOps used for machine learning.
“With deep learning, there aren’t a lot of options, because people are using their own tools,” Tim Negris, the head of marketing for Spell, told EnterpriseAI. But using the company’s newly-unveiled platform, enterprises can now more easily manage their deep learning model training, orchestration, monitoring, reporting, dashboarding and more, he said.
“It is essentially a data and operations infrastructure,” said Negris about the platform. “It has a database that takes care of everything. For regulatory compliance, in things like financial services, this is very important.” Spell captures data about models, then catalogs the models and their results, all while tracking information about who created the models and more, he said.
Negris said the Spell platform has received a wide range of improvements over the last two years while under development and that it is now being introduced to the market formally. Spell has been refined using feedback from early users to drive its maturation and focus on helping make deep learning easier for enterprises to use.
“Up until now we have been in a semi-stealth mode, doing limited promotion and targeted marketing,” said Negris. “But now in the past six months we have added features and completed the range of functions that we want to address. This is just sort of like the coming out party.”
While many companies have been experimenting with AI in their labs or development offices, far fewer enterprises are presently using AI in production today, said Negris. Spell aims to help improve those numbers by removing barriers to adoption so it can be brought into real world business operations, or “operationalized,” with fewer pitfalls.
Spell was co-founded in 2017 by Serkan Piantino – who founded Facebook New York and co-founded Facebook AI Research – and Trey Lawrence, who worked as a technical engineering leader designing silicon chips, PCBs, and firmware, as well as built recommendation systems for e-commerce at eBay and Spring. The company received $15 million in Series A funding in January of 2019.
“The founders were both involved in building out the orchestration and management infrastructure for deep learning at Facebook, eBay and Clarifai,” said Negris. “The aha moment, the thing that dawned on them and brought them together, was the recognition that these big giant companies can build out the infrastructure they need, but for most companies it is just too hard. They felt there was a real opportunity to create an infrastructure management layer underneath the deep learning workflow.”
At its core, Spell automates deep learning workflows from development to training and from deployment to optimization, while strengthening compliance, management and other processes, said Negris.
Adding to its flexibility, Spell can be used by enterprises on-premises or through accounts with multi-cloud and hybrid-cloud infrastructure vendors including Amazon Web Services, Google Cloud Platform and Microsoft Azure, making it cloud-agnostic and allowing users to choose the best implementation situations for their specific needs, said Negris.
“You have workloads that due to regulatory issues and security issues are being migrated down from the cloud to new on-premises gear in the data center,” he said. “And conversely, there is a whole class of workloads that are … in many cases experiments, the initial model design … [that] is being done using on-premises GPUs, that then are migrated to the cloud for the purpose of training an enormous model that might consume many hours of GPU time in many GPUs.”
That ability to choose the right place to run the models is critical and important for users of Spell, he said. “And we have also enabled the ability to transparently stitch together spot instances and on-demand instances and that can now potentially cut your costs in half,” he added.
Spell also includes collaboration features for coordinating work across machine learning and data scientist teams, as well as tools for Kubernetes-based autoscaling and enterprise-grade security, secure sign-on and user/data access controls.
Spell's customers include Akasha, AlphaSense, Cadmium, Condé Nast, Healx, Mulberry, Originate, Quill, Resemble.AI, Whatnot and Square.
Zohaib Ahmed, the CEO of neural text-to-speech vendor Resemble.AI, said in a statement that his company uses Spell to orchestrate its production deep learning payloads so it can focus on creating high-quality models. “The flexibility and reliability that Spell provides helps us scale to build hundreds of models every day," said Ahmed.
Kevin Krewell, an analyst with Tirias Research, said that Spell is apparently part of a trend of vendors that are working on simplifying such tasks for enterprise users.
“You are about to see a wave of companies offering similar MLOps tools,” said Krewell. “For example, Edge Impulse released their AutoML tool EON recently. The ML market is shifting from funding new chip companies to funding new software companies that can bring ML to a wider audience of developers.”
And as Spell and other companies jump in to serve these needs, they will seek out their niches, said Krewell. “Because not all machine learning is the same, there is an opportunity for companies to specialize. Spell's expertise is in deep learning algorithms.”
Another analyst, Chirag Dekate, a research vice president at Gartner, agreed that more and more companies are eyeing the AI, machine learning and deep learning services marketplace.
“Gartner surveys and client engagements indicate an increasing urgency in enterprises to operationalize AI,” said Dekate. “Gartner tracks hundreds of AI startups, with the majority focusing on the different arenas of orchestrating AI. Enterprises are curating AI platforms to orchestrate, automate and scale production-ready AI.”
Spell is looking at the situation in a unique way, said Dekate.
“Spell’s approach is differentiated in that they enable enterprises to leverage a platform approach to operationalizing AI,” he said. “[The] Spell platform enables enterprises to improve productivity of AI teams by exposing underlying on-premises or cloud resources as a shared platform for data scientists.”
The Spell interface is also “versatile in that enterprises leverage their familiarity with Python notebooks to get started and use Spell to manage shared resources on-premises or in the cloud,” he said. “The Spell interface also enables easy tracking of projects, metrics and efficacy of AI pipelines.”