AI Inference Benchmark Bake-off Puts Nvidia on Top
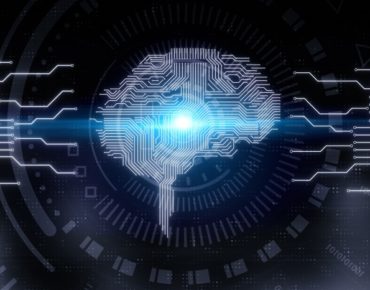
MLPerf.org, the young AI-benchmarking consortium, has issued the first round of results for its inference test suite. Among organizations with submissions were Nvidia, Intel, Alibaba, Supermicro, Google, Huawei, Dell and others. Not bad considering the inference suite (v.5) itself was just introduced in June. Perhaps predictably, GPU powerhouse Nvidia quickly claimed early victory issuing a press release coincident with the MLPerf announcement – “Nvidia today posted the fastest results on new benchmarks measuring the performance of AI inference workloads” – though without much detail.
Actually, navigating the results takes some effort because of the diversity of systems (cloud to servers to mobile devices) and accelerators (GPUs, FPGA, DPS, TPUs, ASICs) covered. Along with the results, MLPerf issued detailed paper explaining the benchmark just as it did for its training test suite. Noteworthy, organizations perform their own testing using the MLPerf suite and results are validated as part of that process. Among accelerators used by systems tested were Nvidia T4s, Arm variants, Habana’s Goya inference processor, Alibaba’s HanGuang, the Hailo8 chip, and others.
Making apples to apples comparisons requires some effort. Different form factors, system sizes, CPU and accelerator counts, frameworks used, all matter. Of the over 500 benchmark results released today, 182 are in the so-called Closed Division (defined below[i]) intended for direct comparison of systems. The results span 44 different systems. The benchmarks show a five-order-of-magnitude difference in performance and a three-order-of-magnitude range in estimated power consumption and range from embedded devices and smartphones to large-scale data center systems. The remaining 429 open results are in the Open Division and show a more diverse range of models, including low precision implementations and alternative models.
As you can see digging out meaningful results will take some work. One of the more accessible comparisons is looking at accelerator performance differences, said David Kanter, co-chair of the MLPerf inference working group, in a pre-briefing with HPCwire, “With respect to the number of accelerators [in a system], one of the things is that [they are] explicitly parallel. So, in some sense, the performance per chip should be the same whether you have 8, 20, 4, or one accelerators. In many respects, I think the way I conceptualize these results is to look at it on a performance per chip basis.”
Wearing his MLPerf neutral hat, Kanter was reluctant to say much about particular entries but did venture that he thought the Alibaba submission was interesting as was an experimental system in the R&D category “that uses low precision, so four-bit integer and it needs to use retraining in order to hit the target accuracy, but I thought it was interesting to see people pushing the limits of what you can do for new forms of quantization.”
It’s best to spend time with the spreadsheet to answer particular questions.
MLPerf first jumped into the AI benchmarking arena with a training test suite in May of 2018 and has issued two rounds of public results since, the most recent in July. Today’s release of inferencing test results, posted on MLPerf.org, was a long-planned step on MLPerf’s roadmap and on schedule.
The v.5 inference test suite, acknowledged Kanter, is modest covering three tasks and uses five well-established models (see chart below):
“We chose tasks that reflect major commercial and research scenarios for a large class of submitters and that capture a broad set of computing motifs. To focus on the realistic rules and testing infrastructure, we selected a minimum-viable-benchmark approach to accelerate the development process. Where possible, we adopted models that were part of the MLPerf Training v0.6 suite, thereby amortizing the benchmark-development effort,” reports MLPerf.
For the rest of this article, please visit sister publication HPCwire.