Nvidia Doubles Down on Medical AI
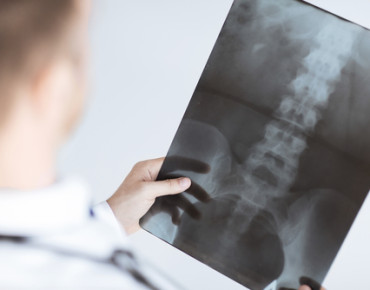
Nvidia is collaborating with medical groups to push GPU-powered AI tools into clinical settings, including radiology and drug discovery.
The GPU leader said Monday (April 8) it will collaborate with the American College of Radiology (ACR) to provide clinicians with its Clara AI tool kit. The partnership would allow radiologists to leverage AI techniques for diagnostic imaging using their own clinical data.
Nvidia (NASDAQ: NVDA) announced a separate AI partnership with the medical consortium, Accelerating Therapeutics for Opportunities, or ATOM, to speed the drug discovery process and reduce the number of false starts. The effort combines “AI, high-performance computing, and advanced experimental technologies intended to help shorten the drug discovery timeline,” the partners said.
The radiology initiative stems from a pilot program. The radiology group will integrate the Clara AI tool kit into a new ACR Data Science Institute, also known as ACR AI-LAB. The free software will be distributed to more than 38,000 ACR members to develop, fine-tune and share AI algorithms. The partners emphasized that patient data would be stored locally.
Among the goals is integrating AI into the radiology workflow. Thousands of algorithms will be needed to analyze radiological images, noted Kimberly Powell, Nvidia’s vice president of healthcare. “Data sets are hard to come by,” Powell added. AI for radiology is a “game changer.”
The AI tool kit includes 13 pre-trained models. “Algorithms typically work best within the sites where they were trained, but those limited training sets are not always representative of the population at large,” said Keith Dreyer, chief data science officer at Partners Healthcare and associate professor of radiology at Harvard Medical School.
“Training AI models on data from diverse sites helps ensure resiliency while reducing algorithm bias, resulting in improved inference across broader populations,” Dreyer added.
The AI tool kit includes libraries for data annotation, model training and deployment. Once deployed, the platform would handle data aggregation, image annotation and pre-processing as well as algorithm transfer and local computing to improve radiological algorithms. Integrating the models into radiological workflows would ultimately democratize AI, Nvidia asserts.
“Radiologists want to get involved in building algorithms that meet their needs,” Powell said on a conference call with reporters.
The collaboration would maintain patient privacy while providing radiologists with a reference architecture that includes software pipelines and other components that can be used to develop native AI tools that can be incorporated in hospital infrastructure, Powell added.
Nvidia also seeks to apply its AI technology to the laborious and expensive drug discovery process. The company is collaborating with ATOM to scale the public-private consortium’s drug discovery platform. The San Francisco-based group is developing a “pre-competitive, pre-clinical platform” that integrates diverse data types with AI and HPC.
The partners noted that high failure rates mean it often takes about six years for new treatments to reach clinical trials. Nvidia’s Powell said the goal of the precision medicine effort is to create data pipelines that can accelerate the drug discovery process.
ATOM’s approach emphasizes GPU-accelerated HPC while using data-driven modeling and generative molecular design to determine drug designs that account for pharmacology, safety and efficacy while attempting to gauge the chances of successful development.
ATOM researchers have so far created a predictive model development pipeline that leverages large datasets “to build and test predictive machine learning models which consider pharmacokinetics, safety, developability and efficacy,” said Barry Selick, an ATOM board member and vice chancellor at the University of California-San Francisco.
NVIDIA’s contribution “will enable this pipeline to be run at increased scale and speed,” Selick said.
“Working with the ATOM team of experts in drug discovery, precision oncology and supercomputing, we will democratize a data-driven, scalable, AI drug discovery workflow that reduces the time and cost to discover new drugs and accelerate the journey to precision medicine,” added Powell.
Related
George Leopold has written about science and technology for more than 30 years, focusing on electronics and aerospace technology. He previously served as executive editor of Electronic Engineering Times. Leopold is the author of "Calculated Risk: The Supersonic Life and Times of Gus Grissom" (Purdue University Press, 2016).