Nvidia Leads Alpha MLPerf AI Benchmarking Round
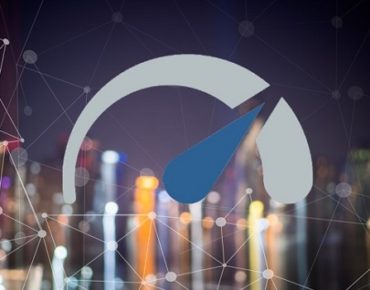
Seven months after the launch of its AI benchmarking suite, the MLPerf consortium is releasing the first round of results based on submissions from Nvidia, Google and Intel. Of the seven benchmarks encompassed in version v0.5 of the would-be benchmarking standard, Nvidia announced that it captured the lead spot in six. Separately, Google (which led the creation of the benchmark) said results show Google Cloud “offers the most accessible scale for machine learning training.”

As HPCwire reported in May, MLPerf is an emerging AI benchmarking suite “for measuring the speed of machine learning software and hardware.” Started by a small group from academia and industry – including Google, Baidu, Intel, AMD, Harvard and Stanford – the project has grown considerably in the last half-year. At last count, the website lists 31 supporting companies: the aforementioned Google, Intel, AMD and Baidu as well as ARM, Nvidia, Cray, Cisco, Microsoft and others (but not IBM or Amazon).
According to the consortium, the training benchmark is defined by a dataset and quality target and also provides a reference implementation for each benchmark that uses a specific model. The following table summarizes the seven benchmarks in version v0.5 of the suite, which spans five categories (image classification, object detection, translation, recommendation and reinforcement learning). Time to train is the main performance metric.

MLPerf Training v0.5 is a benchmark suite (Source: MLPerf)
Nvidia revealed today that its platforms outperformed the competition by up to 5.3x (faster time to results), showing leading single-node and at-scale results for six of the workloads. Nvidia opted not to submit for reinforcement learning network because, as Ian Buck, vice president and general manager of accelerated computing at Nvidia, explained in an advance press briefing, it is for the most part CPU-based and does not have meaningful acceleration in its current form.
Nvidia submitted for all of the six accelerated benchmarks in two categories — single node (testing up to 16 V100 GPUs in the DGX-2H platform) and at-scale (testing in various configurations, up to 640 GPUs).
In a blog post published today, Nvidia stated that “a single DGX-2 node can complete many of these workloads in under twenty minutes. And in the case of our at-scale submission, we’re completing these tasks in under seven minutes in all but one of the tests.”
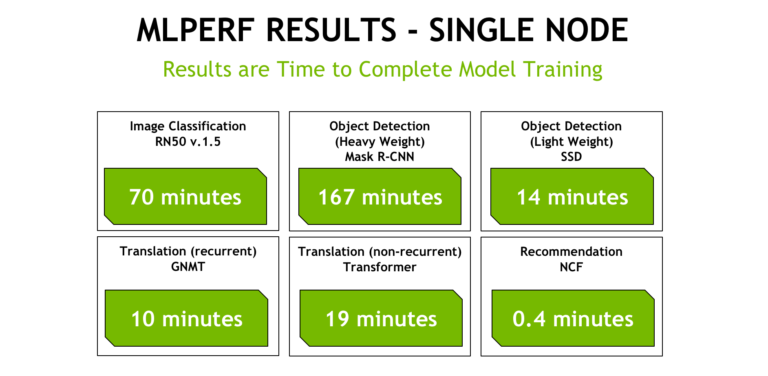
Test Platform: DGX-2H – Dual-Socket Xeon Platinum 8174, 1.5TB system RAM, 16 x 32 GB Tesla V100 SXM-3 GPUs connected via NVSwitch (Source: Nvidia, see endnotes for details)
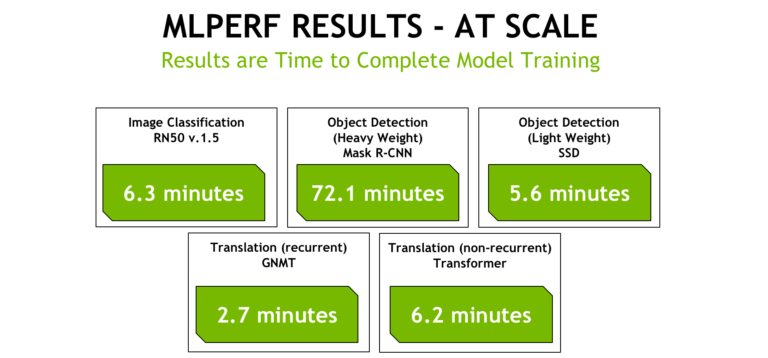
Test Platform: For Image Classification and Translation (non-recurrent), DGX-1V Cluster. For Object Detection (Heavy Weight) and Object Detection (Light Weight), Translation (recurrent) DGX-2H Cluster. Each DGX-1V, Dual-Socket Xeon E5- 2698 V4, 512GB system RAM, 8 x 16 GB Tesla V100 SXM-2 GPUs. Each DGX-2H, Dual-Socket Xeon Platinum 8174, 1.5TB system RAM, 16 x 32 GB Tesla V100 SXM-3 GPUs connected via NVSwitch. (Source: Nvidia, see endnotes for details)
While there are faster ResNet50 competitions out there, they aren’t under the standard MLPerf guidelines, Nvidia told us.
“By improving and delivering on the full-stack optimization and our performance at scale, we decrease training times, which makes research and deployment of AI faster and we improve the cost efficiency,” said Ian Buck in the press briefing. “If I take a DGX Station and look at its value over four years, it’s roughly $1.50/hr, so a little over $6 to train a ResNet50.” Buck added that Titan RTX, announced last week with a list price of $2,499, comes out to just over $2.00 to train a single ResNet50.
Speaking to the value of the “industry’s first comprehensive AI benchmark” and what that means for customers, Buck stated: “Nvidia is no stranger to benchmarks; we certainly have them in the graphics space, we have them in the supercomputing space and we now have them as well in the AI world. Providing a common benchmark, a common set of rules as long as it’s appropriately governed can provide perspective to customers and the rest of the community on the state of everyone’s solution. It also provides a nice common platform for people to innovate, to measure innovation and help companies move the ball forward in improving the performance.”
Google also took time to promote its results today in a blog post, claiming Google Cloud “offers the most accessible scale for machine learning training” and “a 19 percent TPU performance advantage on a chip-to-chip basis.”
“The results show Google Cloud’s TPUs (Tensor Processing Units) and TPU Pods as leading systems for training machine learning models at scale, based on competitive performance across several MLPerf tests,” wrote Urs Hölzle, Senior Vice President of Technical Infrastructure, Google.
“For data scientists, ML practitioners, and researchers, building on-premise GPU clusters for training is capital-intensive and time-consuming — it’s much simpler to access both GPU and TPU infrastructure on Google Cloud,” said Hölzle.
This graphic from Google compares absolute training times for Nvidia’s DGX-2 machine, containing 16 V100 GPUs, with results using 1/64th of a TPU v3 Pod (16 TPU v3 chips used for training and 4 TPU v2 chips used for evaluation). The three benchmarks shown are image classification (ResNet-50), object detection (SSD), and neural machine translation (NMT).

Training time comparison between 1/64th of a TPU v3 Pod (16 TPU v3 chips used for training, plus four separate Cloud TPU v2 chips used for evaluation) and an Nvidia DGX-2 (16 V100 GPUs) (Source: Google Cloud)
The inaugural MLPerf testing only had three submitters: Nvidia, Google and Intel. All submitted for the closed division, which compares hardware platforms or software frameworks on an “apples-to-apples” basis. There were no submissions for the open division, which allows any ML approach that can reach the target quality and is intended to foster innovation. See results here: https://mlperf.org/results/
Noted on its Github page, “MLPerf v0.5.0 is the ‘alpha’ release of an agile benchmark, and the benchmark is still evolving based on feedback from the community.” Changes under consideration include “raising target quality, adopting a standard batch-size-to-hyperparameter table, scaling up some benchmarks (especially recommendation), and adding new benchmarks.” The current suite is limited to training workloads, but according to Nvidia, there are plans to add inference-focused benchmarks. The consortium is working on releasing interim versions of the suite (v0.5.1 and v0.5.2) in the first half of 2019 with a full version 1.0 release planned for the third quarter of 2019.
Related
With over a decade’s experience covering the HPC space, Tiffany Trader is one of the preeminent voices reporting on advanced scale computing today.