AI Translation of Online Sarcasm for Sales Research. No Kidding
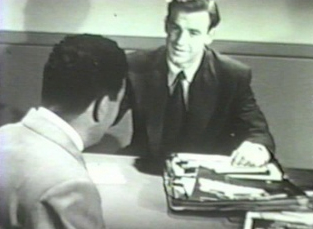
“I don’t hate Mondays at all.” “This sugar-free, gluten-free quinoa cake is exactly what I wanted for dessert.” “I am totally happy skipping my vacation to work a few extra hours for free.”
Sarcasm like this is commonplace in conversation and on social media. Most of us are adept at detecting it. But take sarcasm out of traditional verbal conversation settings and place it in the flat modern playing field of text and email exchange, and the detection of acerbic irony becomes problematic.
What if AI could be used to detect sarcasm in plain text and translate it for readers? Could it have impact on business and sales? My research team at Israel’s Technion University thought so, so we went ahead and built it.
The potential applications are significant. In addition to my academic work I work as a data scientist where this research is being used to help sales teams struggling to identify means and methods for successful agent-to-customer conversations. These salespeople often find themselves wading through mountains of transcribed conversations, many of which can present hidden stumbling blocks in the form of sarcastic phrases or disingenuous remarks. But a company that utilizes a sarcasm interpretation generator as part of its transcription package can surmount this hurdle, saving both time and effort on the part of its sales agents eager to learn how and why their calls succeeded or failed.
The goal of my research team at the university was novel: Using AI, we would be able to identify sarcastic utterances on Twitter and instantly translate those utterances into non-sarcastic phrases carrying the same meaning. We chose Twitter as our environment for studying for two reasons: Sarcasm is prevalent on this forum, and we were able to sort phrases by the key “#sarcasm.”
With the reverse-meaning properties of sarcasm in mind, we designed the Sarcasm SIGN (Sarcasm Sentimental Interpretation GeNerator), an algorithm which capitalizes on sentiment words to produce sarcasm interpretations. Over time, the algorithm can be applied at much larger scales, including those critical to big data, and various simulation modeling scenarios that touch upon language and communication within the realms of English online conversation.
Our study spanned six months of sarcasm on the web. Using the Twitter API, we collected tweets marked with #sarcasm posted between January and June of 2016, sorted and filtered them to eliminate any tweets that were unoriginal (retweets), tweets that contained URLs, memes or other images, and tweets written in a language other than English.
We were left with some 3,000 text-only sarcastic tweets as our dataset, and we got to work.
A team of 10 workers, all of them with active social media presences themselves (to ensure baseline familiarity with the medium) and professional backgrounds in comedy and literature paraphrasing (to ensure they were adept at the task of hand) created, together, five non-sarcastic translations/interpretations of each sarcastic text that made up our dataset. For example, given the tweet “how I love Mondays. #sarcasm,” we created parallel interpretations, including “how I hate Mondays” and “I really hate Mondays.”
The next step was to teach the machine to translate the sentences on its own. Our method was to employ automatic machine translation evaluation metrics, using two widely-used MT approaches: phrase-based MT and neural MT. We checked the machine’s work based on a) fluency of translation: how readable/natural sounding is the machine-generated translation?; b) adequacy of translation: how accurate is it in capturing the meaning of the original sarcastic text? and c) sentiment: is the feeling described by the translated phrase the same as that expressed in the original sarcastic tweet?
Our algorithm begins by identifying and clustering sentiment words according to semantic relatedness. Each sentiment word is replaced with its cluster number and the transformed data is fed into an MT system, both at training and test phases.
Beyond the sales use case discussed earlier, there are other more general applications for societal gain. Men and women on the autism spectrum often struggle with interpersonal relationships, and an inability to detect sarcasm speech or insincerity in conversation poses one of their most significant communicative hurdles. SIGN, when applied to text conversations, Facebook messenger interchanges and translations of emails and other electronic correspondence, could, in the future, open up significant opportunities for those on the autism spectrum to bridge this conversational gap by simultaneously translating hard-to-read phrases into those that are more easily comprehended.
Lotem Peled is a data scientist and natural language processing researcher at Gong.io.