Machine Learning Fuels Scientific Skepticism
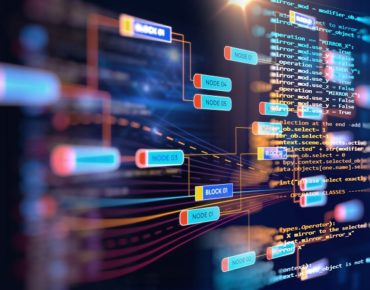
Scientists are raising red flags about the accuracy and reproducibility of conclusions drawn by machine learning frameworks. Among the remedies are developing new ML systems that can question their own predictions, show their work and reproduce results.
Concerns about the efficacy of early machine learning predictions in disciplines ranging from astronomy to medicine were noted by Rice University statistician Genevera Allen during last week’s annual meeting of the American Association for the Advancement of Science.
Allen said brittle ML frameworks are often flawed since they are designed to come up with some kind of prediction, often failing to account for scientific uncertainties. "A lot of these techniques are designed to always make a prediction," Allen said. "They never come back with 'I don't know,' or 'I didn't discover anything,' because they aren't made to."
Allen, an associate professor of statistics, computer science and electrical and computer engineering at Rice, questions whether scientific discoveries based on the application of machine learning techniques to large data sets can be trusted. "The answer in many situations is probably, 'Not without checking,'” Allen said.
Read the full story here at sister web site Datanami.
Related
George Leopold has written about science and technology for more than 30 years, focusing on electronics and aerospace technology. He previously served as executive editor of Electronic Engineering Times. Leopold is the author of "Calculated Risk: The Supersonic Life and Times of Gus Grissom" (Purdue University Press, 2016).